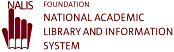
Authors:
Todorov, Apostol; Stoykova; Zlatev
Title:
IMPROVING SIGNAL STRENGTH ESTIMATION IN IOT USING WI-FI NETWORK PERFORMANCE DATA
Date of Issue:
2023
Is Part of:
APPLIED RESEARCHES IN TECHNICS, TECHNOLOGIES AND EDUCATION
Publisher:
Тракийски университет - Стара Загора
Identifiers:
1314-8796 [issn]
Type:
Article
Language:
eng
Subject:
WiFi networks; signal strength prediction; regression modeling; additional signal features; accuracy improvement; network performance analysis; computational simulations; educational buildings
Abstract:
This study aims to improve signal strength prediction in WiFi networks by incorporating
additional signal characteristics and using advanced regression modeling techniques. The research
builds on existing work and offers a more accurate approach for predicting signal strength in WiFi
networks. Using the RReliefF method, the influence of the main characteristics of the wireless signal on
its quality has been established. Data were reduced using the Principal Components Analysis method.
They were used in creating a complex predictive model for signal quality in a WiFi network. By analyzing
key characteristics of wireless signals, the study achieved an increased prediction accuracy of 94%.
The proposed regression model overcomes the previous limitations and offers a reliable method for
predicting signal strength. The research goes beyond graphical representations and focuses on
regression modeling to present more accurate estimates. The study includes computational simulations
and real-world measurements to assess signal strength in an educational building. Furthermore, the
research addresses the coverage issues of wireless sensor networks within WiFi networks, aiming to
provide effective solutions to existing challenges. By overcoming the limitations, the study contributes
to the overall improvement of WiFi networks. The obtained results have practical implications for network
planning, optimization, wireless sensor networks, and network performance analysis. The research also
paves the way for future studies to explore machine learning techniques, cross-layer analysis, and
energy efficiency considerations in the context of WiFi networks. Overall, this study offers valuable
information for predicting signal strength and improving WiFi network performance.